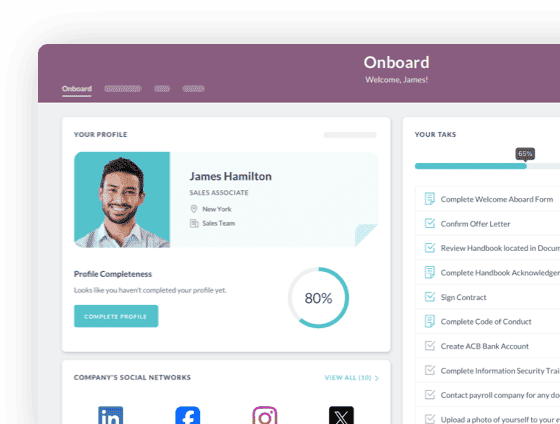
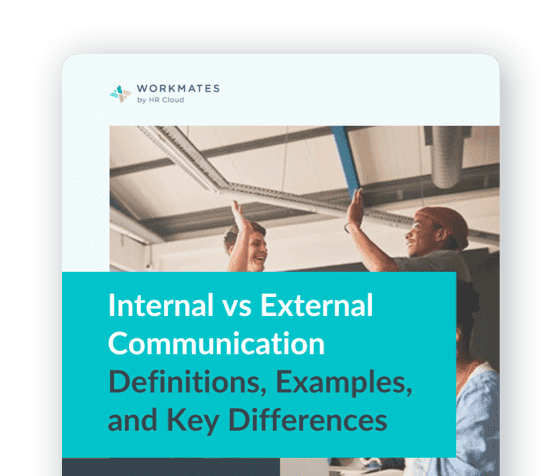
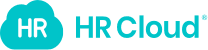
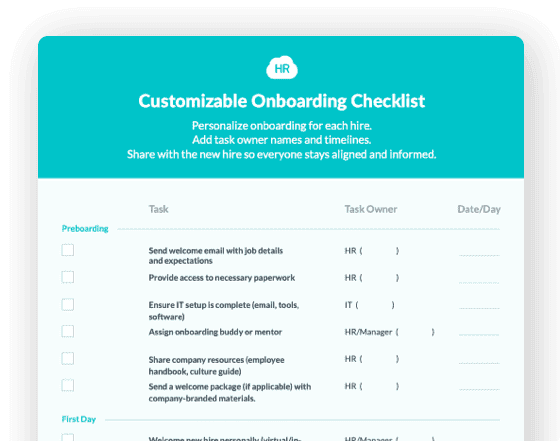
Following the digitalization of the job market, including a growing reliance on free job posting sites for e-recruitment and the emergence of remote working models, the reach of the recruiting and job-hunting process has massively expanded. As a result, the traditional pool of candidates and job ads has turned into a vast ocean of potential recruits and open positions.
Crafting a winning job post is one of the strategies for attracting top talents. For example, LinkedIn reports that 95 job applications are submitted every second on its platform and that each week, 50 million users access it to search for jobs.
This certainly represents a tremendous opportunity for both hiring managers and job seekers. However, the ability to post your jobs on a platform with a virtually unlimited outreach would end up being counterproductive without proper tools to filter the most relevant options, as recruiters screening hundreds of candidates are well aware of. And that's where machine learning-based recommendation systems for recruitment come into play.
The role of recommendation systems
Recommendation systems for recruitment are powered by computer algorithms and designed to route professionals toward an open position matching their aspirations and expertise, or companies toward suitable candidates based on their business requirements. These tools, currently implemented by all major employment-oriented online platforms and social media, can perform a variety of HR-related duties, such as:
-
Segmenting professionals into sub-categories or archetypes depending on their profile information (country, previous work experience, skills, certifications, and more) and behavioral patterns (such as likes, shares, comments, and browsing history).
-
Segmenting employers and their respective job listings data based on the industry, location, current staff, open positions, requirements, and more.
-
Providing employers with a list of potential candidates, sorted by relevance, while targeting job seekers with tailored recommendations regarding the most interesting job opportunities.
-
Suggesting the best certifications and highlighting the core strengths to compete for a certain position.
Thanks to their segmentation and matching capabilities, recommendation systems for recruitment can act as a catalyst to connect professionals and corporations on the job market. Furthermore, they can easily mitigate the so-called information overload suffered by HR departments when flooded with thousands (or millions, as for multinational corporations like Google) of applications. For example, a recruitment proposal can help you revolutionize the way you segment and attract top talent, making your hiring process efficient and engaging like never before.
However, the above wouldn't be possible without the essential contribution of machine learning algorithms. So, let’s better frame this technology and its actual application in this field.
Post Jobs, Evaluate Candidates,
and Hire the Best with Our
Applicant Tracking System
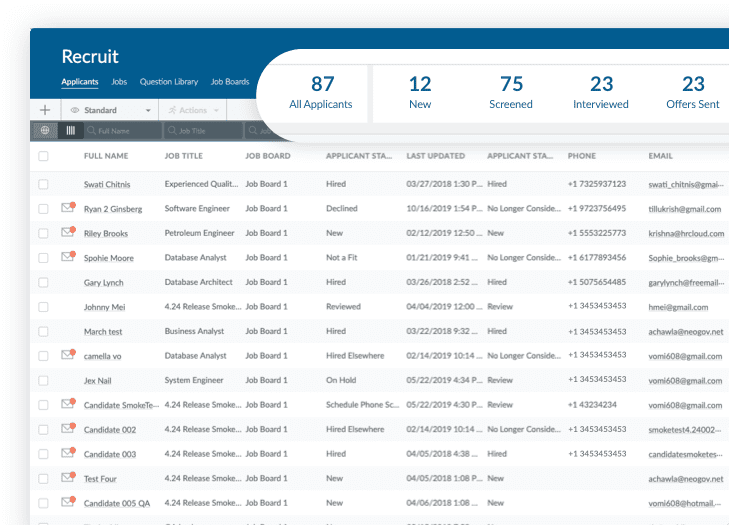
Why apply machine learning in e-recruitment
Nowadays, any advanced recommendation engine for recruitment relies on machine learning to peer into data and provide relevant suggestions. Specifically, this subset of AI strictly related to data science and analytics concerns the creation of self-learning algorithms that can process data, spot key variables and recurring patterns among them, and create models representing such relationships.
As the name suggests, a core strength of machine learning algorithms is their ability to learn and improve through experience. Specifically, they can identify more and more correlations as they process new data and refine their models to provide a more truthful portrait of the scenarios and dynamics examined. Once a model has been trained with enough data, it will be leveraged by a machine learning system to process new datasets and provide valuable insights or predictions.
For example, the ML algorithm powering a recommendation engine for recruitment can be "fed" with datasets collected through a professional networking platform. After proper training, the algorithm may observe that having a certain qualification or trait in the user profile generally results in a greater chance of being hired by a specific type of organization, be it a large IT corporation or a small business. Thus, the engine will start recommending properly qualified professionals to that employer and vice versa.
ML algorithms for recruiting
How the aforementioned routing process takes place depends on the algorithms used and the recommendation system design approach. Regarding the first point, researchers have tested several machine learning algorithms whose performance could actually range according to the operational scenario, including the number of platform users and relevant metrics to consider.
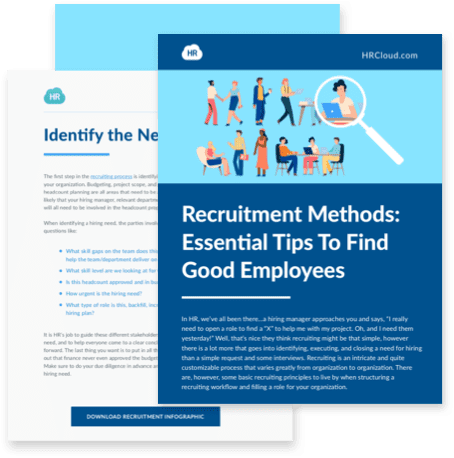
To learn more about recruitment methods, download our ebook now.
Among the algorithms typically implemented in this kind of software solution, we may count naive Bayes classifiers, K nearest neighbors, random forest, support vector classifiers, logistic regression, and gradient-boosted decision trees. The latter, for example, have also been adopted by LinkedIn for its well-known Recruiter feature.
As for the design approach, this aspect of recommendation systems deserves its own section.
Types of recommendation systems for recruitment
Similarly to their eCommerce-focused versions, recommender systems for recruitment can be built according to three major design approaches defining the underlying mechanisms for providing recommendations.
Collaborative filtering
E-recruitment recommendation engines embracing this approach base their suggestions on similarities among users. Indeed, they recommend candidates that other companies with similar requirements have already expressed an interest in or suggest jobs that other professionals with common traits have previously applied for.
Thanks to their ability to accurately frame users based on context-aware variables and identify non-linear relations between them, collaborative filtering engines currently represent the most successful segment of the recommendation system market, so much so that major hiring platforms such as LinkedIn and Indeed rely on similar tools.
Content-based filtering
The focus, in this case, is not on the relations among similar users, but on the convergence between similar items. In a real-world recruiting scenario, the “items” are online job ads or candidate profiles. The recommendation system will examine job seekers' preferences for certain ads and recommend new job listings sharing similar features with the previous ones. At the same time, it will assess recruiters’ interest in specific candidate archetypes and suggest other profiles with similar characteristics.
This implies the analysis of features from user profiles, curricula, and cover letters (such as age, language, skills, education, and more) and job descriptions (including employer, industry, country, responsibilities, etc.). The aforementioned information is typically extracted by identifying specific keywords and concepts via natural language processing technologies.
“Interim Healthcare SLC needed HR technology, and we’re pleased with the results we’ve gained from HR Cloud’s solutions for recruiting, onboarding, and employee engagement. Yet it’s an opportunity for all Interim franchises. It would be so great if each franchise owner could implement similar solutions to replace legacy systems that might not work as well as they should.”
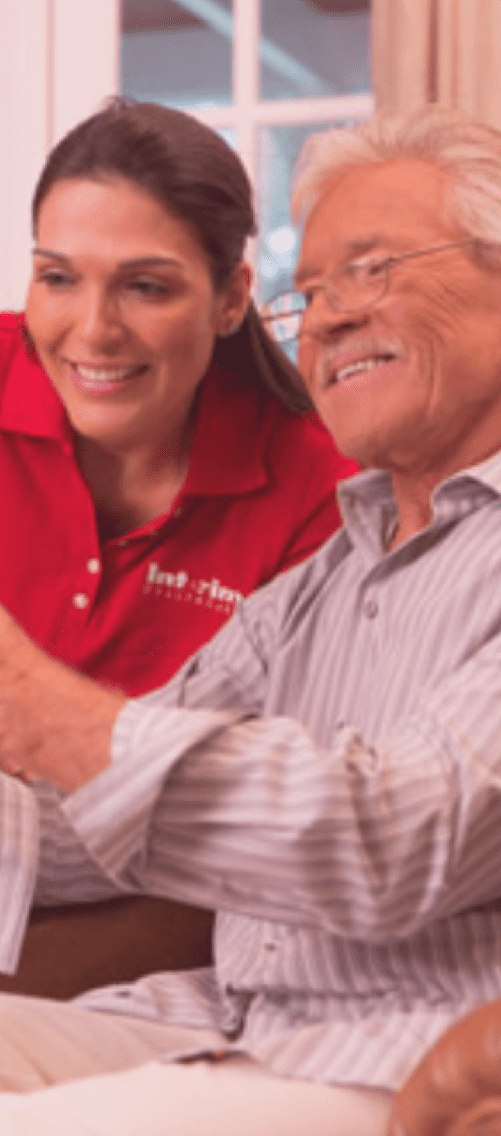
Hybrid approach
This hot trend in recommendation system design is aimed at combining the key strengths of both approaches at the cost of creating a more complex architecture and requiring more computing power. Such systems rely on different hybridization techniques to provide job and candidate recommendations, including the creation of a single processing pipeline in which the result of the collaborative part becomes the input for the content-based phase.
Reshaping the job market with algorithms
While human relationships and networking will always remain staples of job search and recruiting, we can expect a growing role played by recommendation systems and machine learning algorithms in our professional lives.
But if it's true that online recruitment and the possibility to post jobs for free on global platforms have turned the job market into an ocean of opportunities, ML-powered recommendation systems will act as a beacon to guide ships to a safe harbor, or skilled professionals toward a company ready to value their expertise.
Author Bio:
This article is written by our marketing team at HR Cloud. HR Cloud is a leading provider of HR solutions, including recruiting, onboarding, employee engagement, and intranet software. Our aim is to help your company improve employee engagement, and employee productivity, and to save you valuable time!
Keep Reading
Retention Reset: How to Keep Your Best Talent in 2025
More employees are walking away from their jobs in 2025 not just for better pay, but for
The Most Common Hiring Mistakes in the Healthcare Industry
The hiring process can be tedious in the healthcare industry, and it may be tempting to
Remote Onboarding Success Stories
When it comes to onboarding new hires, many companies understand the value and benefits
Like What You Hear?
We'd love to chat with you more about how HR Cloud® can support your business's HR needs. Book Your Free Demo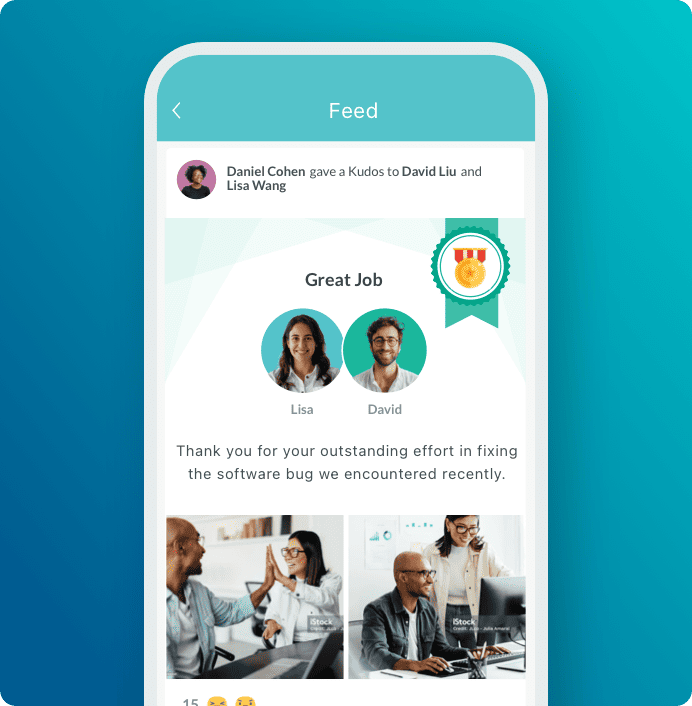
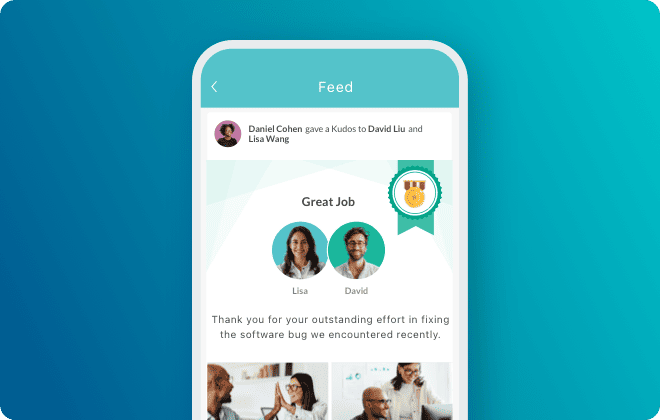
See Workmates in action
Your culture upgrade starts here. Take a quick tour or book a live demo.
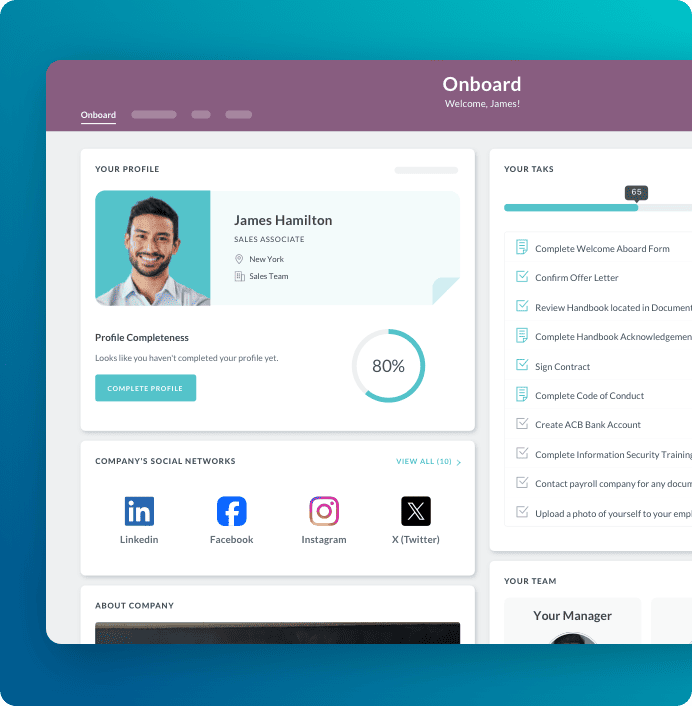
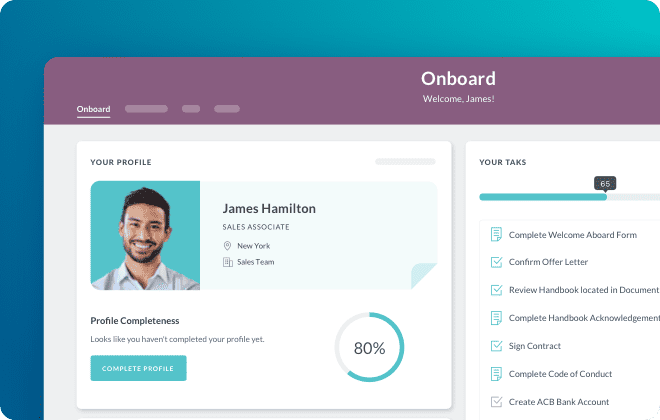
Cut onboarding time by 70%
See how growing teams streamline onboarding and save hours for every new hire.